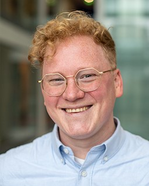
We’re delighted that one of VISION’s core researchers, Dr Niels Blom, has been awarded a prestigious UK Data Service (UKDS) Fellowship.
The award will be used to improve the reach and impact of Niels’ research on violence and abuse and its relationship with job loss, health, and wellbeing. He is using several UKDS datasets, including the UK Longitudinal Household Survey and the Crime Survey for England Wales, to understand the link between violence, particularly intimate partner violence, and its socioeconomic, wellbeing, and health impact.
For more information about Niels, his work, and what he hopes to get out of the Fellowship scheme, see his blog on the UKDS website.
The UKDS is funded by the UKRI and houses the largest collection of economic, social and population data in the UK. Its Data Impact Fellowship scheme is for early career researchers in the academic or the voluntary, community, and social enterprise (VSCE) sector. The focus in 2023 is on research in poverty, deprivation, the cost of living crisis, housing and homelessness, using data in the UK Data Service collection. The purpose of the programme is to support impact activities stemming from data-enhanced work.
For further information on the UK Data Service please see: UK Data Service
To read Niels’ blog please see: UK Data Service Data Impact Fellows 2023: Niels Blom – Data Impact blog
Or contact Niels at niels.blom@city.ac.uk
Photo by Alina Grubnyak on Unsplash